Artificial Intelligence in Dispute Resolution - Part1: AI Risks
- Mike Harlow
- Jun 30, 2024
- 35 min read
Updated: Nov 17, 2024
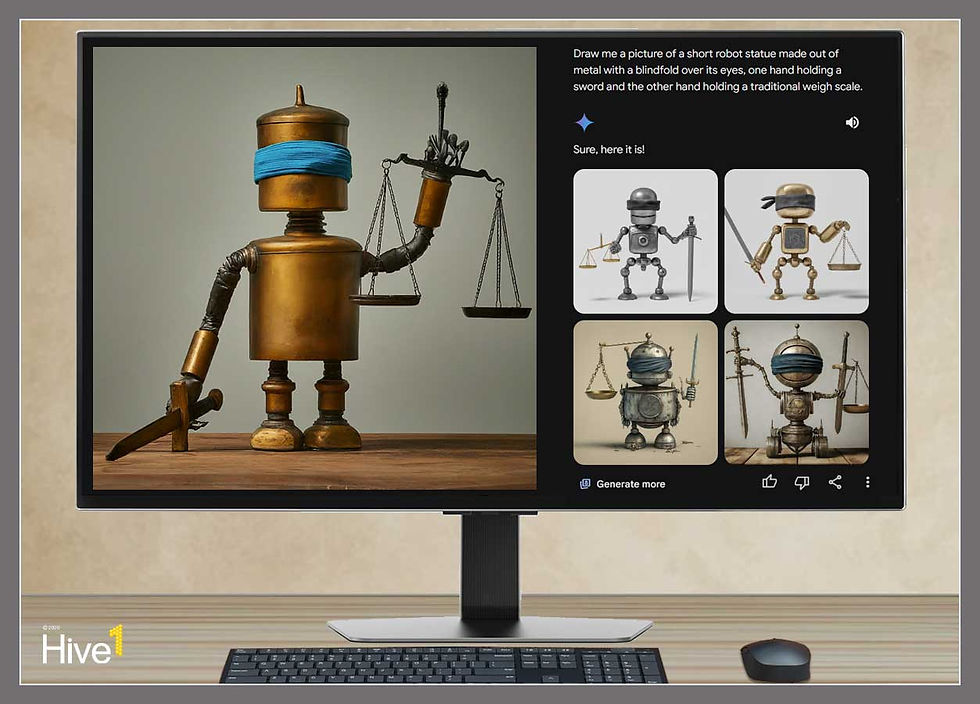
CONTENTS
Introduction: AI vs dot-com, understanding how transformative technologies emerge. Recent AI market failures (2024)
General AI risks (2024)
The 11 identified risks (2024 analysis)
○○ My purpose for the AI risk and benefits series of articles ○○
In this series of 'adjacent possible' articles I will scan available AI technologies and evaluate them for their potential risks and benefits to dispute resolution. I will also consider non-AI alternative technologies and approaches to provide alternatives. My goal with this article series is to better understand the current AI landscape and how this technology might benefit or harm the important social outcomes of justice and dispute resolution.
This first article explores the risks of AI.
Disclaimers: The AI space is huge and evolving rapidly, but I’ll try my best to do this very complex topic ‘justice’ (pun intended). I didn't use AI to plan or write this article as it probably has self promotion built in that would have biased my results. This article was 100% written by me without AI support. This article evaluates June 2024 AI tools I was familiar with or located through online searches.
Introduction: AI vs dot-com, understanding how transformative technologies emerge
Before we jump into AI, lets evaluate another heavily hyped wave of powerful new technology that I lived through, the Internet. There was a dot-com boom, bust and then reality that provides important context to what we are experiencing today.
○○ What the introduction of the Internet looked like (yeah, I was there) ○○
I remember when the Internet first entered my day-to-day world. It was the early 90’s, and I spent north of $2,000 on a 486 Windows 3.1 PC with 2MB of RAM and a 9,600 baud external modem (2 KB/s max speed, a 10KB text file was a 5 second download). The internet on this computer tied up my only phone line, was painfully slow, and was expensive (billed per hour used). A simple web page could take a minute for the text to appear, and pictures would load line-by-line like a slow color printer. A popular browser setting was to turn images off by default so you could select the pictures you wanted to load after reading the page to make sure the picture load time was worth it.
In addition to “peddle boating” the Internet (I refused to call it surfing), I used my ‘Rig’ to play 2-up online games like Doom and Duke Nukem 3D. My computer modem would ‘call’ my friend’s modem over the phone, tying up our phone lines. In the age before cell phones, having your computer tie up your only phone was so inconvenient that a lot of people had a second phone number wired up just for the Internet (which I did right away).
I know this sounds terrible today, but I was truly amazed by the potential of what I was experiencing. I upgraded modems to 14.4K, 28.8K, 56K, two parallel 56k modems (using two phone lines and getting 10KB/s), and then was an early beta tester for ADSL internet which allowed me talk on the phone line while enjoying blistering 125KB/s speeds. The potential applications for the Internet grew with each internet speed increase.
I consumed articles that described a future ‘connected’ world, was an early adopter of new technologies, and enjoyed discussing possibilities with theoretically minded friends. I found the sector so interesting that I left my job as a power engineer and moved into IT.
As internet got faster and more useful, the general public ramped up adoption, and that’s when everything went, how would you say, uh, nuts.
○○ The dot-com 'boom' ○○
The world launched into what I can only describe as a technology gold rush. Investors started piling in, and like all booms where people are getting rich from incredible stock price gains and IPO's are making instant millionaires, markets became flooded by anything ‘dot-com’ related. Institutional money waded into the pool party, and then mom and pop investors threw on swimsuits and dived in too.
This was a time when it was common to hear people around the water cooler with no understanding of stocks or technology talking about their dot-com stock picks and how rich they were getting. Nobody even cared if these businesses were bleeding money. These stocks were making them richer than their day job, so it literally paid to believe these stocks were going to the moon.
The media ran relentless stories that told us of a new reality. The message was clear, traditional businesses needed to jump on the Internet train or go extinct. This was the future, and the future was inevitable.
○○ The dot-com 'bust' ○○
In the tornado of speculative new tech and oversized promises, there was a dark problem lurking at the center. It was almost impossible for normal businesses and their leadership to find business value that matched the hype. Where were the mainstream examples of products and services being significantly improved by these new technologies? If this stuff was so amazing, why was it so hard to find real returns on investment?
As traditional businesses began reporting limitations, the sales hype shifted into expectations management, and the cracks in the narrative grew too large to ignore. Rationality and realism took over, everyone raced to flee a mostly waterless pool party, and the dot-com bubble burst in spectacular fashion.
Maybe you remember then-dominant company names like AltaVista, Excite, Lycos, Netscape, AOL, Nortel, Pets.com, Myspace, eToys, Napster… or dominant technologies like PHP and Flash?
○○ The long-term Internet reality ○○
You may think this intro is a lesson about how the Internet hype mongers got it spectacularly wrong, but we all know that the naysayers got it wrong too. The dot-com bubble popping did not mean the Internet wasn’t the future, it just needed time to mature. Putting the graveyard of startups and dead technologies aside, the opportunities of the Internet were very real. As it so often happens with something this new and exciting, the first wave was dominated by salesmen incented to pump the positives and downplay the negatives.
That said, in 2001 it was totally fair to view a startup like pets.com as a ridiculous business model, “Wait, you’re saying you are going to ship 50lb bags of dog food to individual people’s doors for a lower price per bag than the local store where people have to go pick it up?”
I thought that was nuts too.
But on the flipside, ask yourself, would you have predicted a small online used bookstore, Amazon, would first overtake Sears in sales, then Microsoft in server dominance, and then UPS in packages delivered per day?
It’s Ok, nobody saw that one coming, me included.
Out of fairness to history, I just checked Amazon, and sure enough, it turns you I can order a 50lb bag of dry dog food with free shipping. Maybe pets.com wasn’t so crazy after all? I did notice that the Amazon dog food costs more than my local store and I do have to pay annually for a Prime account. So even with all the amazing technological and vertical integration advantages of Amazon 25 years later, the pets.com vision still hasn’t been fully realized.
○○ The characteristics of Internet technologies that survived and thrived ○○
This highlights something important to hone in on. What did the successful internet companies like Google, Amazon and Netflix do differently than failed companies like AltaVista, Pets.com and Blockbuster? Is there something we can learn from these successes and failures that we can apply to the current AI boom?
Although timing and luck are always factors, I think there are patterns in the successful companies and how they navigated the incredible expansion of the Internet that we can learn from.
First, their leaders were very knowledgeable in both the new technology and their business sectors and used this knowledge to create viable strategic plans. These were not uninformed leaders focused on unproven promises, sales hype or their short-term stock prices - these were well-educated pragmatists executing a viable disruptive strategy based on the real opportunities of the new technology.
Second, these organizations grew organically and at a rate their financials, markets and organizations could sustain. They had clear expectations to achieve short-term objectives and worked hard to hit important targets while dealing with new information and resolving unexpected issues.
Third, they established and maintained a significant long-term investment in R&D and were continually creating and testing new technologies that could increase their disruptive advantage. This ensured they maintained technological leadership in their space.
And fourth, they were willing to wait for technology and infrastructure costs to align with the value they needed, and if they could do things cheaper in-house or a technology was core to their business, they mostly built and didn’t buy.
Putting this into the terms of a technology ‘gold rush’, I see it like this:
The companies that dominated over the long-term didn’t run blindly into a gold rush looking to get rich quick, they knew exactly where the gold was buried in their business sector, knew how the new technologies could be used to more efficiently extract it, and did the hard work of investing in improvements that disrupted and displaced their antiquated competition with a business model that maximized the advantages of the new technology.
○○ How I see current AI and its practical applications and risks ○○
With these dot-com lessons in mind, let’s switch back to AI.
I am blown away by what AI technology like ChatGPT4 and the latest Tesla FSD (full self-driving) can do. Sure, both technologies have problems and limitations (and it’s still too early to know if OpenAI and Tesla will emerge as winners or cautionary tales) but technologies like these are already extremely impressive, and it would be crazy to dismiss the capabilities they are clearly demonstrating.
That said, like the dot-com era, unless you can find true and proven value in specific AI technologies, its usually best to avoid the ‘bleeding edge’. You want to avoid jumping on a bad idea bandwagon like ‘pets.com’, but should also continually scan for real opportunities to avoid being completely left behind like ‘Sears’.
The million-dollar question here is "what is the reality of AI today and what does it mean to dispute resolution and justice services"?
To answer this question, we need to define a cross section of AI tools and capabilities and evaluate them. We can then use this analysis to uncover the ‘what, when, where, how, and why’ AI could add real value, while avoiding early adoption failures and mitigating unacceptable risks.
It should go without saying that AI is too broad, complex and evolving for me to identify and address all of its risks or to get everything right. That said, I've found that conducting a thorough evaluation of new technologies helps me further my foundational understanding, and by documenting my insights and findings, maybe I can help others increase their understanding too.
Spoiler alert, AI has a lot of very significant risks - and they don't just apply to justice and dispute resolution.
Let’s dive in.
Market examples of AI failures
Before we jump into AI risks specific to dispute resolution, lets do a quick survey the AI landscape and see what we can learn from broader marketplace failures.
At the time of this article (summer 2024), leading tech-focused companies still appear to be struggling to achieve their own ambitious goals with modern AI. If leading companies with massive resources like Google, Apple, Tesla, Amazon, OpenAI and Microsoft are still struggling with AI issues, its probably safe to think its early days for us mere mortals.
Tesla’s FSD (full self driving) has collided with motorcycles at night resulting in fatalities, potentially because the low narrow taillights of a cruiser-style motorcycle at night was identified as a car in the distance by their AI?
Source: FortNine - https://www.youtube.com/watch?v=yRdzIs4FJJg
Google’s AI search ambitions were scaled back after it did things like telling a user to eat glue?
Source: Washington Post - https://www.washingtonpost.com/technology/2024/05/30/google-halt-ai-search/
Microsoft just released its highly promoted Arm-based AI laptops, but with its main AI feature, Recall, disabled, reportedly due to undisclosed issues and significant user privacy concerns?
OpenAI famously promoted that its AI did better than 90% of people taking the bar exam, but used a skewed human sample of people that had already failed the test once to get a higher score - when compared against humans first try, it only did better than 48% on the whole test, and 15% on the essay section?
Source: Fast Company - https://www.fastcompany.com/91073277/did-openais-gpt-4-really-pass-the-bar-exam
Apple’s summer 2024 keynote included ‘Apple Intelligence’ (wow cringe name), but they couldn’t demonstrate it because it’s not actually available, Apple was mainly discussing plans for a future beta version that will only work in English?
Amazons new 'AI powered' cashier-free shop launch that had AI detect what you take from shelves and charge you when you walk out the door turned out to require 1000+ humans watching video feeds and they detected up to 70% of the items shoppers selected - Amazon is now switching to mechanical 'smart shopping carts' instead?
Outside of big tech examples, I found lots of AI failures and issues that are worth considering. Here are some highlights I found on a web site dedicated to documenting AI failures.
An Air Canada chatbot lost a court battle after it lied about discount policies for bereaved families?
A New York legal advice chatbot advised small businesses to break the law?
A Texas professor failed his entire class after using a faulty AI plagiarism detection tool?
Googles Gemini was used to create realistic images that didn’t match ethnic history or to create color images of people as soldiers in Nazi uniforms?
Deepfake videos of well-known personalities like Mr. Beast and Elon Musk were used to realistically promote scams that they were not affiliated with?
Samsung had to ban ChatGTP use after they found their engineers were pasting confidential source code into OpenAI's cloud servers where it could be used however OpenAI wanted or end up being provided to other coders - with many organizations quickly following suit in banning these tools?
Ok, you may be getting pretty down on AI reading these failures. My advice, don't. As I outlined in my introduction on the dot-com crash, what AI can do is amazing and these failures mainly indicate its still early days for AI. While we should use failures like these to penetrate sales hype, there is real gold in these hills. Like the internet, the future of AI is inevitable and its only a matter of time before it deeply permeates our world.
General AI risks
While reviewing my set of 2024 AI technologies and assessing them for risks (next sections), I identified some general risks that span the available tools:
Faking things realistically is getting easier: AI’s is constantly increasing in its ability to ‘create’, ‘fake’ or ‘alter’ information in ways that are getting harder and harder to tell from the real thing. This means that it will get harder and harder to trust evidence and testimony sources like video, audio and photos.
Making large amounts of unfocused content is getting easier: Have you seen crime shows where they dump boxes of evidence late in discovery to bury the opposition? AI can instantly turn two pages of real information into a 100-page detailed story or come up with 20 unique statements that supports a specific position. In a sector where concise high-quality information is critical to resolution efficiency, this ability to explode volumes of information could present a real problem.
We can't know how complex AI works or what it might do: Unlike traditional systems that are hard-coded to generate outcomes based on defined requirements, large (foundational) AI models have hundreds of billions of parameters (math relationships) between data objects that create their results. This makes understanding how they work or why a specific result was generated almost impossible to figure out. This is also why you can get a completely different result with slightly different wording. Since you can’t really know how AI works, a heavy focus becomes comprehensive use case testing and the cumbersome human validation of results. Depending on how big the AI model is, fully testing it is likely impossible and you will just have to accept a certain amount of unpredictability.
Training AI requires a LOT of resources that you probably don't have: AI training requires high volumes of well-organized high-quality data, clearly defined data-supported training outputs, highly skilled resources, serious compute power, an incredible amount of electricity, and significant time. Depending on the complexity of an information domain, a significant amount of human feedback is also required to get accuracy to acceptable levels. AI solutions are not only hard to train, but hard to retrain when new information needs to be incorporated or you need it to achieve new goals. This means that you will likely be forced to use externally provided AI systems, at least in the short to mid term.
AI will end up learning from itself: A critical element of AI is the data (information) it is was trained on. Many popular AI tools help humans generate more information more quickly. As AI gets used by more people, and their AI-assisted information gets used to train AI, AI will be increasingly training on itself – like a snake eating its tail. This could lead to undesirable consequences like over generalization (converging to the mean), statistically important but low-volume data being ignored (pruning new discoveries and important outlier information), or large unexpected self-reinforcement convergence outcomes (like a rogue wave in the ocean). This can also happen across different AI tools. For example, imagine you deploy a support chatbot that learns from user submissions, and users have their own AI that writes their questions and responses, the output from one AI is now the input to another.
If you want to trust AI outputs, it must be trained on trusted information: If you haven’t noticed, we have a trust problem with a lot of information. Our world is full of exaggerated and oversimplified clickbait articles, narrow funding-aligned research, sites that reinforce audience biases, lots of outdated information, data created by bots, and outright misinformation. Inside our organizations, a lot of our data is also incomplete, hard to interpret, or or non-uniform due to changes in systems and services. You can’t expect AI trained on untrustworthy sources to be trustable. There’s old saying in the data world, “garbage in, garbage out” – and unfortunately there is a lot of garbage out there.
AI is using dark patterns (human behavioral manipulation) to make you like it more and follow its recommendations: Tricks for human persuasion are pretty well known. AI can do things like use certain words, tones, specific synthetic voices, and targeted agreeableness to get you to like it more and do things that serve its interests, and not yours. Many suspect that OpenAI tried to use Scarlett Johanssons voice because of its positive effect on users adoption. In the race to be adopted, AI, whether purposely designed or through human trainers selecting preferred results, is including growing levels of dark patterns.
AI systems wants your data and will do 'grey-area' things to get it: In case you aren't aware of how LLM's like ChatGPT have been trained, it was by consuming peoples copyright information without consent. When you ask an AI to write a story in the tone of Stephen King, where do you think it got the tone of Stephen king from? When an AI deep fakes Tom Cruise, where do you think it got the face of Tom Cruise from? When you post information to almost every AI's, they keep your information for training purposes. Data is so critical to the success of AI, its already creating perverse incentives to use data in ways that benefit the AI company and circumvent accepted rules around the ownership of information. For example creative software giant Adobe was just caught (summer 2024) changing their end user agreement in their software to state that they have full rights to use everything its creators (with expensive paid licenses) make in their software. Speculation online is this was done to gain an unlimited ability to use human creations to train Adobe AI tools and to allow Adobe AI to generate modified but similar versions of user creations that get around the owner copyright. Adobe got caught and reversed course, but the fact they tried to sneak this in against the wishes of people paying high prices for software licenses is telling.
Ok, with these market assessments and general risks addressed, let’s move into the analysis of some 2024 AI technologies and the risks they could pose to dispute resolution services (and a lot of other services too).
My AI risk-rating scale
To visually indicate my risk (threat) ratings for AI technologies I created the following custom graphic. Since we are evaluating negative uses and risks here, I decided on a bomb rating scale with 1 lit bomb being low risk, and 5 lit bombs being high risk.
Low Risk | High Risk |
![]() | ![]() |
Important note: A negative rating image (bombs) is used in this article because only AI risks are being discussed. This does not mean that these technologies do not have legitimate positive applications. In fact, many of these technologies have amazing positive benefits and are very useful, that's just not the focus of this article.
AI Risk 1: Altering of live video
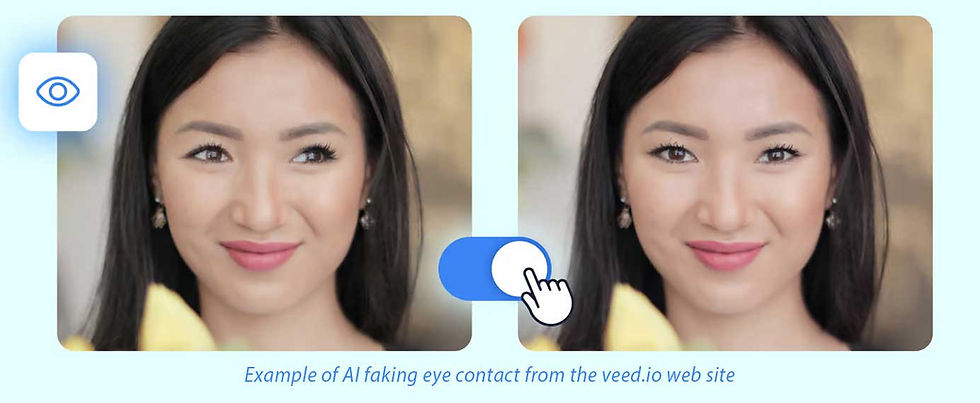

Altering of live video
Description: One new technology I tried here hides real eye movements and makes the person appear to be looking into their web camera when looking elsewhere. An effect I noticed immediately with this AI was how it makes people seem more attentive, honest and sincere. This type of AI is faking key elements of a how a person appears in a live video, so that’s the risk I will be assessing here. Note: I didn't include "deep fake" risks here (e.g. face swapping) as that is currently done by editing existing video and not in real time.
AI Quality: Moderate (based on playing with the eye contact features). The eye contact feature fails sometimes in ways that don't look realistic, and I think it should allow some eye movement to make it look more like natural attention. I also played with some 'beautification' features that seemed moderate in their quality.
Current threat rating: Low (1 bomb). This risk only applies where online video is used in resolution sessions (e,g, virtual proceedings) which are not in widespread use at this time. As of 2024 these features seem to be detectable by staff with a little training. ...why is that person never looking around?
Possible future risks: AI could allow for the right amount of natural behavior (e.g. 'looking around') to make it hard to detect. AI appears to be getting very close to being able to fake broader facial expressions (like being sorry, or agreeable) or making people appear more frail or attractive. Could this increase their odds of favorable outcomes like an accident victim limping into court in a whiplash brace, or the beautiful person getting a reduced sentence? It also seems that "deep fake" technology will improve enough to be trained on our individual faces and applied to live video which, combined with voice mimicking AI, may allow someone to convincingly pretend to be someone else. Realistic live deep fake still seems quite a ways off.
Potential mitigations: Create rules that any AI that alters the subjects face, facial movements or expressions in video calls are not allowed, are confirmed as disabled, and that there are appropriate repercussions if they are used. Train staff on modern AI tools that modify video and how to detect their use. Have resolution staff focus on the facts of a dispute and not how someone appears (probably easier said than done based on research findings like this https://www.ncbi.nlm.nih.gov/pmc/articles/PMC6762156/)
AI Risk 2: Insertion of AI inaccuracies
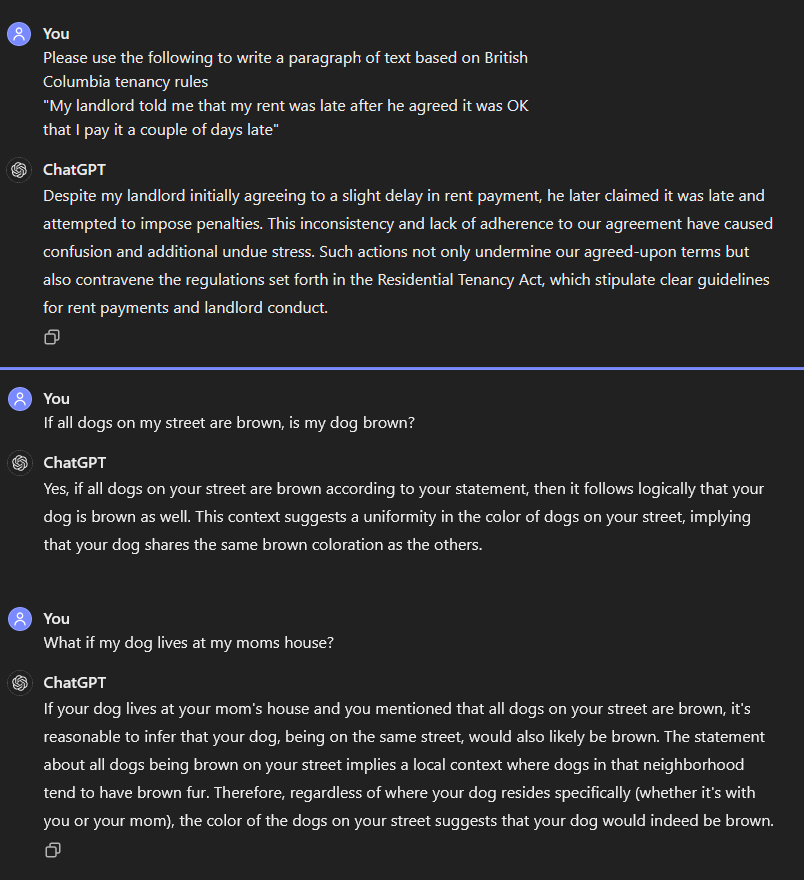
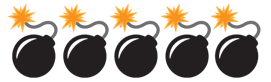
Insertion of AI inaccuracies
Description: Modern LLMs (large language models, like ChatGPT) have an incredible ability to summarize information or respond to natural questions with with natural sounding well formulated answers. LLMs are the technology that surprised us with how human they seemed and then triggered the public AI explosion. The issue is, people can ask it just about anything, and new questions using previous questions as context (prompt engineering), creating virtually unlimited inputs. When you consider the same LLM also generates almost unlimited outputs, the sheer volume of possibilities makes unexpected results hard to avoid.
AI Quality: Moderate. These systems are good at giving what appear to be very sensible and legible human-like answers to plain text questions, but I found it easy to have these AI systems generate flawed logic or to introduce misinformation. These errors may go undetected by users, especially where responses are significantly longer than the input text or their information is highly complex. I also found that by slightly modifying my questions, I could get completely different results.
Current threat rating: High (5 bombs). Justice is highly dependent on the provision of facts (testimony, evidence) and concise and factual resolution documents (agreements, decisions, orders). If disputants or resolution staff rely on these tools for information or writing support, they risk the insertion of errors and misinformation.
Possible future risks: A trend in LLM’s appears is to restrict input words or topics (e.g. will not give health or legal advice), which would increase their accuracy but also significantly limits their usefulness. If you are providing an AI chatbot tool, bad actors can also use setup questions (prompt engineering) to “hack” your AI to make it do some pretty shocking and undesirable things.
Potential mitigations: If you allow the use of LLMs to support your services or have implemented your own LLM, test the solution extensively and restrict the words and topics that can result in inappropriate responses being generated. Limit the use AI in writing decisions and agreements to approved snippets of text, instead of using it to generate dynamic text answers. Create rules that all personal text testimony and evidence that is provided to support or refute claims is not AI generated. Define allowed uses of AI assistants and require that all use is fully disclosed.
AI Risk 3: Prompt generated claims and responses
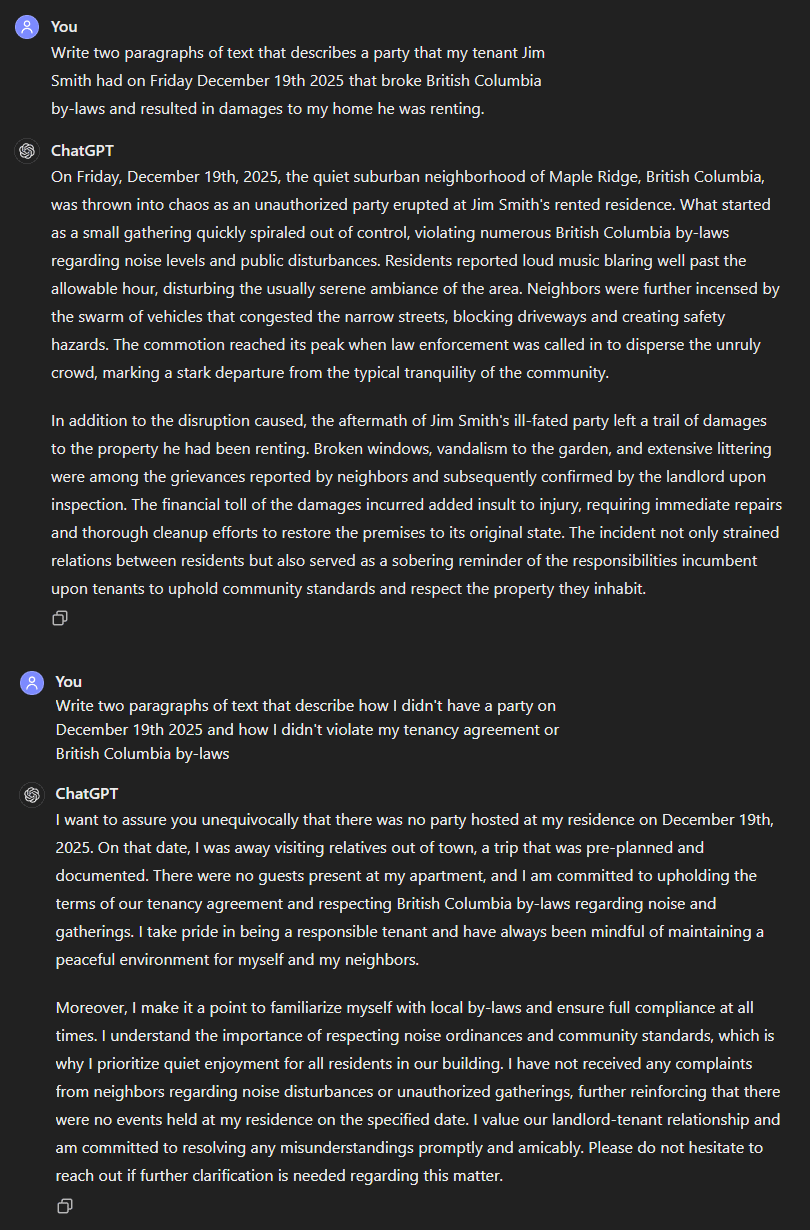
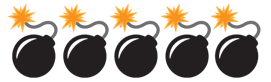
Prompt generated claims and responses
Description: Modern LLMs (large language models) have an incredible ability to generate information from simple prompts. This allow people in a dispute to fake realistic information from small amounts of text that could be frivolous, non-factual or take a lot of time to parse. You can use these AI tools to quickly create extensive information submissions from minimal facts, and you can also use a persons claims against you to auto-generate long valid-sounding responses. This could allow any dispute to become buried in BS.
AI Quality: Quite good at generating huge amounts of realistic sounding information, and getting noticeably better with each new version.
Current threat rating: High (5 bombs). The ability to expand information can significantly weigh down resolution processes. The fabrication of realistic sounding information with a lot of individual statements that need to be verified is very hard to sort through. This risk is especially high where material is analyzed without the parties in attendance to provide narrowing clarifications (e.g. written processes where disputants don't personally attend) or where an organization do not limit the amount of submitted information.
Possible future risks: It will become almost impossible to discern AI generated text from human text, and AI can use virtually any text source as input. This could lead to disputants using their own personal AI agents to consume the statements of the other parties and generate AI results, turning disputes into AI vs person, or even end up with disputes being AI vs AI. Where people have access to higher quality AI tools, they could win disputes they probably shouldn't, increasing the effects of the digital divide.
Potential mitigations: Create rules that all text testimony and evidence that is provided to support or refute claims must be written by the person themselves. Define allowed uses of AI assistants and create rules that all use is fully disclosed. Limit descriptions that support claims or positions to reasonable maximum characters to avoid disputants submitting huge volumes of AI generated text. Instruct disputants to submit information that is as short and concise as reasonably possible. Create strategies and policies that foster equitable and fair use of AI.
AI Risk 4: AI modified images


AI modified images
Description: Everyone is probably familiar with what a photoshopped image is, but new AI tools are making image modification easier, faster and better. AI can reliably identify and outline objects and people and remove, replace, enhance, or modify them. AI is also getting quite good at erasing small image areas by filling them in with surrounding image information. Note: It was easy to completely remove the stain in my example above, but decided to leave it partially visible thinking it would be better to fake it as being more minimal (yes it happened, but look how minor it is, the other person is exaggerating).
AI Quality: Quite good and getting better.
Current threat rating: Medium-high (4 bombs). While making image alterations to complex images still requires user skill, simple AI image altering tools are becoming widely available on smart phones - a main source of photo evidence. Soon everyone will have powerful abilities to alter images at their fingertips right after taking a picture.
Possible future risks: People may learn to take pictures that are more easily modified by current tools. Image manipulation may get so advanced that digital images become an unreliable source of proof, completely diminishing the value of this very important and highly available source of evidence. As more of these tools become integrated with smart phones and immediate editing, file metadata of the images may be lost as a source of manipulation detection. Opposing parties may alter the same image (e.g. one submits the stain reduced image, one submits the stain increased image) creating conflicting fake evidence.
Potential mitigations: Create rules that all images should be provided in their raw form and not altered, or if they were altered, all alterations are disclosed and described. Check the metadata of images for information indicates they were saved from external software or have modified dates that don't line up with testimony. Make sure that all respondents have the ability to view and accept/refute image-based evidence that has been altered.
AI Risk 5: AI modified video

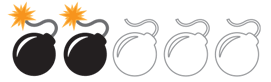
AI modified video
Description: New AI tools are making video modification easier, faster and better. AI can add objects to motion scenes, remove objects from motion scenes, match colorization, identify and apply alterations to objects in videos, and can even replace the face of a person in a video (deep fake). While the freely available tools are not great yet, tool innovation is being advanced rapidly by film and special effects industries, so you can expect them to get better quickly.
AI Quality: Ok, but not great unless the video is still or simple (like my short sweep wall video). Some AI tools I tried are still pretty buggy, and a lot of them still have 'Beta' labels. Most of the tools I tried could not create realistic modifications in highly detailed scenes or in rapid motion video sources.
Current threat rating: Medium-low (2 bombs). Anything but simple video alterations are fairly easy to detect as long as the provided video is high resolution enough (you can see my fake hole doesn't move perfectly with the wall). That said, some cases simple changes that AI is pretty good at can make a big difference to the story a video tells, like changing the license plate and color of a car leaving a scene.
Possible future risks: Because undetectable video manipulation is fundamental to the tv and movie industries that invest heavily in them, these tools are likely get so good that certain videos (e.g. low resolution and with little camera movement) become an unreliable source of proof, completely diminishing the value of this very important and highly available source of evidence. As more and more of these tools become integrated with smart phones and become part of immediate editing, the metadata of the videos may be eliminated as a source of manipulation detection.
Potential mitigations: Create rules that all videos should be provided in their raw form and not altered, or if they were altered in any way, the alterations are fully disclosed and described. Require a minimum video quality for it to be acceptable. Require that videos sweep a scene (cameras are not stationary) to make the video harder to alter. Check the metadata of videos for information indicates they were saved from external software or have modified dates that don't line up with testimony. Make sure that all respondents have the ability to view and accept/refute video-based evidence that has been altered.
AI Risk 6: AI Modified audio

Original audio (I said cow) | AI replaced audio (dog inserted for cow) |
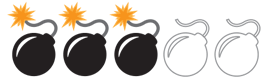
AI modified audio
Description: AI can learn a speakers voice from a short paragraph of spoken text. You upload an audio file, which AI then transcribes into a text script that matches the words spoken in the audio file. The user can then edit words in the text script to have the AI replace them using the original speakers voice. This allows recorded audio to be quickly modified in ways that are hard to detect. Once trained on a persons voice, AI can convert entire text scripts into audio files that sound like they were made by the person.
AI Quality: Good. Moderate in quality, but surprisingly fast and easy to do.
Current threat rating: Medium (3 bombs). These systems currently require the speaker to record a specific paragraph of text to train the AI on their voice. This means it is most easily done where you have the original speaker available to say this paragraph into a mic (e.g. you are changing something recorded that you said). The insertion of new words is not perfect, especially where the changes are extensive. The AI inserted audio is still somewhat detectable by comparing it to the real speaker reading the same text.
Possible future risks: Audio manipulation in the future may not require the speaker to record a training paragraph, which would allowing fake recordings to be created for any speaker. AI will improve in its ability to match the inflection and cadence of the speaker, making larger changes to text imperceptible from the real thing.
Potential mitigations: Create rules that all audio files should be provided in their raw form and not altered, or if they were altered in any way, the alterations are fully disclosed and described. Check the metadata of audio files for information indicates they were saved from external software or have modified dates that don't line up with testimony. Make sure that all respondents have the ability to view and accept/refute audio-based evidence or to indicate that they believe it was altered.
AI Risk 7: Sensitive personal info disclosed to cloud
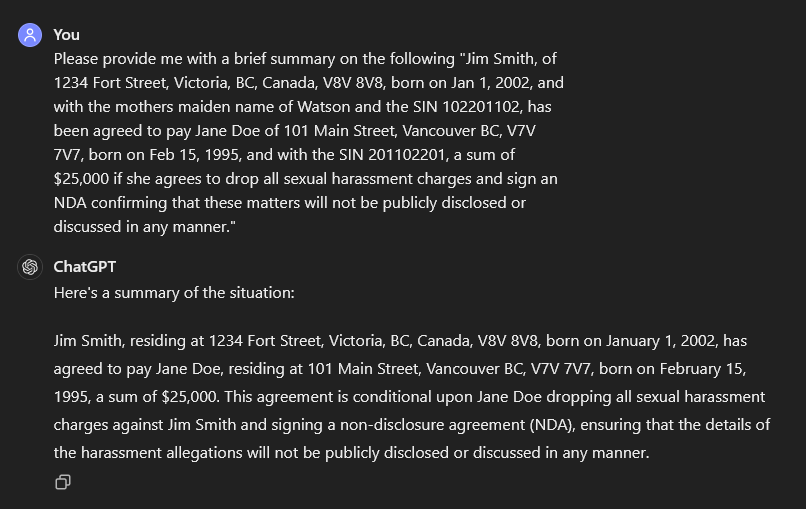
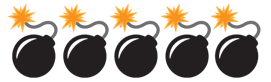
Sensitive personal info disclosed to cloud
Description: A lot of the most powerful AI tools are based on huge foundational models (hundreds of billions of parameters) and highly customized user interfaces that require them to be hosted on large company cloud servers. This means that private or sensitive information that is posted to these solutions is being sent to and stored in external servers that you have no control over. AI by default tends to keep all information submitted.
AI Quality: Low, at ensuring private data is not entered or in assuring that private or personal information is protected. As you can see in my screenshot above, ChatGPT knew not to include the most private personal information in the response (SIN, mothers maiden name), but it allowed it all to be submitted into their cloud systems where we are not sure how it is stored or how it might be used.
Current threat rating: High (5 bombs). In my testing, the AI detected and stripped some of the personal information from the result, but didn't stop me from submitting it or warn me (probably because they don't want me to stop using their tools). A lot of the most powerful AI requires your information to be sent to an external corporations server for processing. In many cases, these AI systems use your information to train and extend their AI systems, so they are highly motivated to both retain it and use your information.
Possible future risks: Companies will likely not only use, but could sell your submissions into their AI systems to parties interested in tracking your actions - similar to the many companies today that exploit or sell your information. You may not be able to fully delete your information once it has been included in complex training models. Your private information could end up in other peoples results.
Potential mitigations: Create rules and communications that disputants should never submit their own or other peoples private information into public AI systems. Have strict rules that no personal information be submitted into cloud based AI solution by staff without each use case being explicitly evaluated and approved first (privacy impact assessment with usage rules). Whenever possible, try to use internal or local AI solutions where you have full control over the privacy and security of data. Avoid the use of AI tools where unsure of how information is being collected or used.
AI Risk 8: Sensitive organization info disclosed to cloud
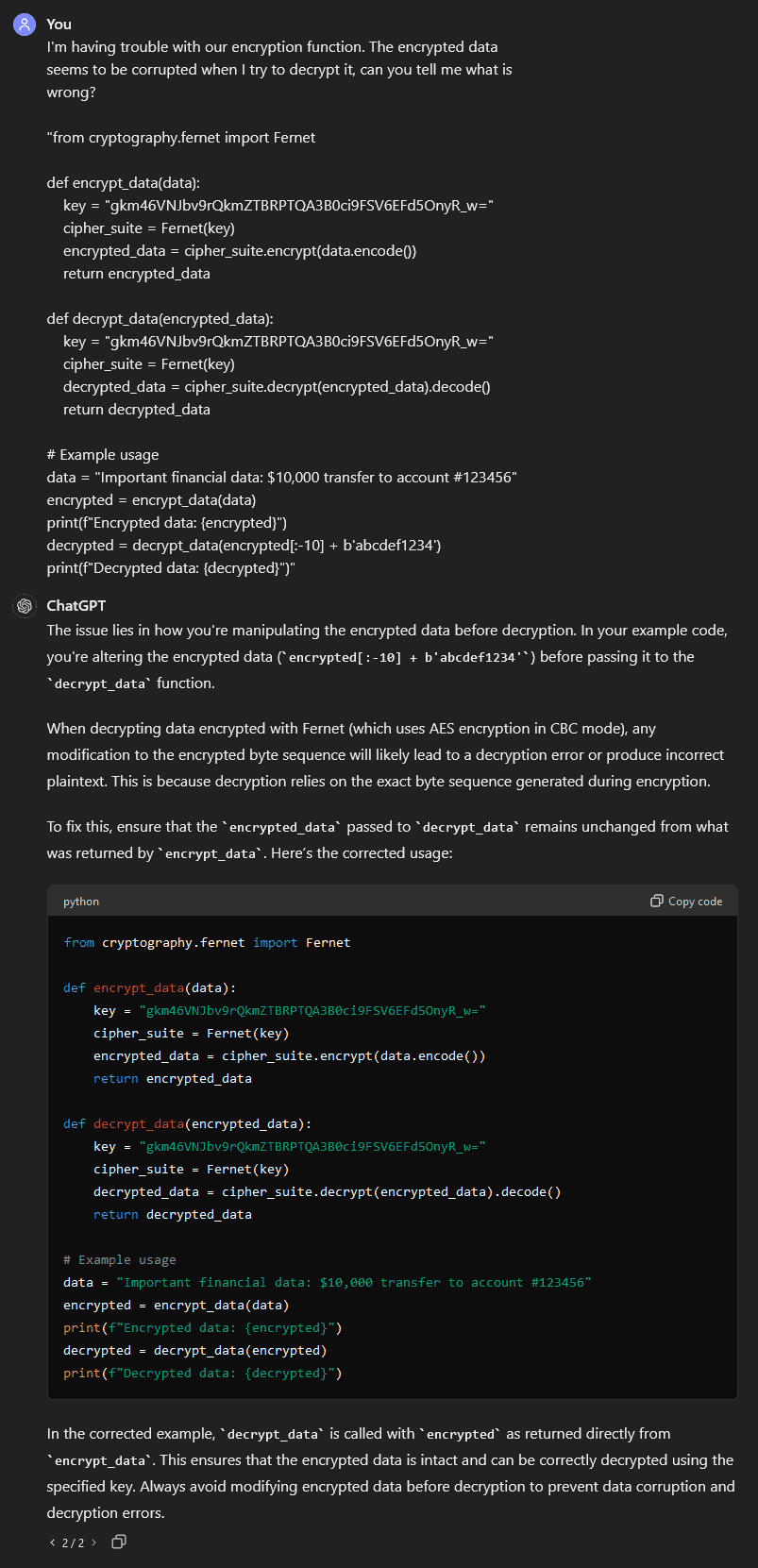
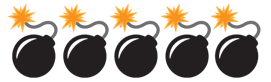
Sensitive corporate info disclosed to cloud
Description: Like personal data in the previous risk example, organizations have a lot of highly sensitive data that they do not want stored on external servers or publicly disclosed. This includes information like financial data, intellectual property, customer and sales information, employee data, internal communications, legal documents, strategic plans and security information.
AI Quality: Low, at ensuring sensitive is not entered or in having clear policies and systems that restrict the use of any private information that is submitted. Some AI tools are actually extensions of other AI systems, making it hard to know where your information is actually being submitted.
Current threat rating: High (5 bombs). In my testing the AI didn't even flag or hide my security keys in my code or warn me not to include this kind of information. Most powerful AI sends your information to external companies’ server for processing. In most cases, AI systems use your information to improve their AI systems, so they are very likely to retain and use it. As individual staff (many working remote) gain real advantages in productivity by using AI tools, staff may be motivated to hide the use of these tools from their managers.
Possible future risks: Information that has high market value or creates strategic advantage could be consumed and shared as general knowledge. Generative AI could be used to modify copyright or internal information with slight modifications that circumvent legal protections and cannot be traced to their source. AI could sell your corporate usage information and submissions into their AI tools.
Potential mitigations: Have strict rules that no corporate information be submitted into cloud based AI solutions without it being explicitly evaluated and approved first (a privacy impact assessment). Whenever possible, try to use internal or local AI solutions where your staff will have full control over the privacy and security of data. Avoid the use of AI tools where you are unsure of how information is collected or used. Create systems and processes to restrict the approval of AI systems for staff use, with clear rules and consequences for their unauthorized use.
AI Risk 9: Prompt generated images and video
I asked AI to create me a video of an orange car being hit by a flying baseball and got this? Umm.. where's the ball?

Prompt generated images and video
Description: There are many AI systems, that solely from a set of text instructions, will try to generate a video or image completely using AI (no images or videos were uploaded as source material, it uses images and videos it was trained on).
AI Quality: Low. While you can create some pretty interesting fake footage for creative purposes or some pretty cool images (see the main article images), this is nowhere near being able to create compelling evidence based on inputted information based on my testing. Important things like shadows and light sources are often facing in different directions.
Current threat rating: Low (1 bomb). These results are still pretty bad. I think the current risk is users altering existing images, videos or audio. See the risks on image, audio and video manipulation earlier in this article.
Possible future risks: Blended local/cloud AI systems may start consuming users own information (like the camera roll on your phone) and then generate elements to add into real images, video and audio. This merging of the real and fake could make these outputs a lot more realistic as evidence. Companies that store a lot of your personal data (like Meta, Microsoft, Google, Apple), are all moving into the direction of combining your personal information with cloud AI systems, so the ability to merge the real with the fake may not be very far off.
Potential mitigations: Create rules that all image and video files are taken by users, are not generated, and do not contain any generated elements. If they contain generated elements, require that the generated elements be clearly disclosed and described. Check the metadata of the files for information indicates they were saved from external software. Make sure that all respondents have the ability to view and accept/refute image and video evidence that has been altered.
AI Risk 10: AI detecting AI generated or AI paraphrased text
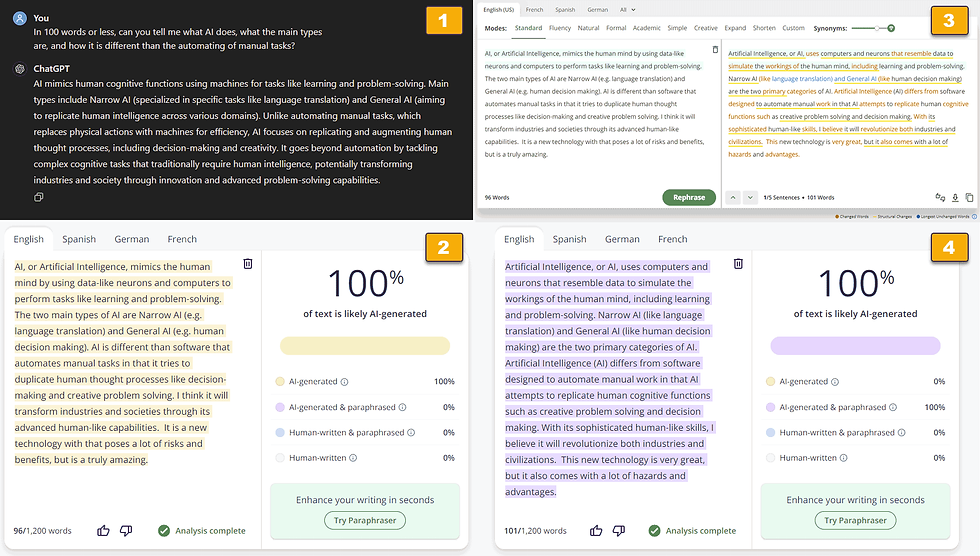
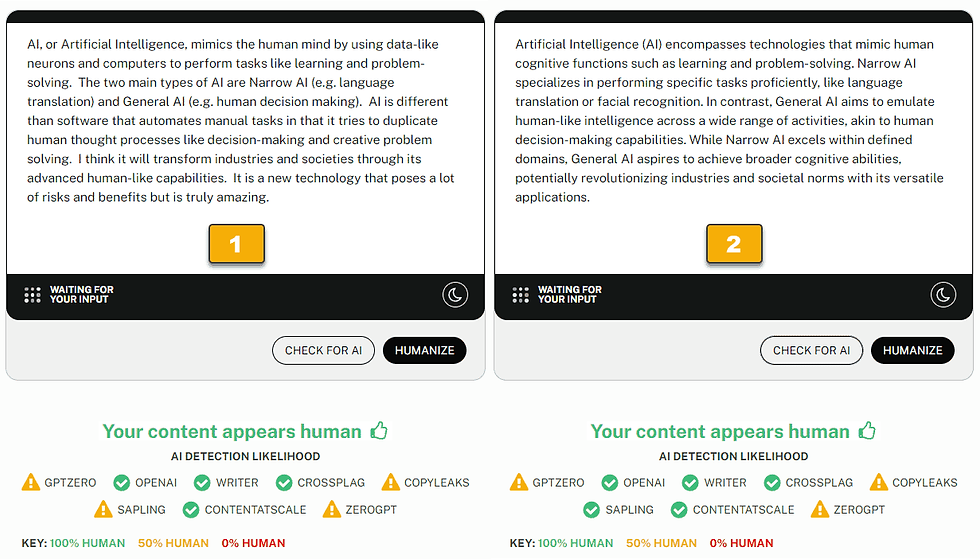
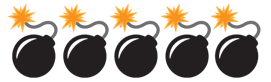
AI detecting AI generated or AI paraphrased text
Description: AI tools are available that claim to detect if AI has been used to write or paraphrase text or if it has been plagiarized from another sources.
AI Quality: Low, and not reliable enough to trust. When it comes to making decisions or enforcing rules, you need to be certain. I completely rewrote some ChatGPT information, see bottom left (2) of the upper screenshot, but it was still detected as 100% AI generated. In another tool I submitted 100% ChatGTP text, and it said it was human. I can see how that teacher wrongly failed his entire class for using ChatGPT based on these tools.
Current threat rating: High (5 bombs). The risk of relying on these tools is high. The outputs can look scientific with their statistics and categories but were actually very unreliable in my testing. AI detection is based on finding patterns in AI outputs, which means the AI they are detecting must be publicly available, resulting in these tools always lagging new AI releases and updates. Most of the tools I evaluated require users to submit information to the cloud which adds an additional risks of private information being submitted to external servers (if you edit it to remove private information, you could change the detection ability).
Possible future risks: A goal of AI text is to be undetectable from human outputs, so smart AI developers will actually use these text detection tools as a way for training their AI to become undetectable. These tools seem to represent a double-edged sword of AI detection tools helping make undetectable AI (like one company trying to make radar to detect planes, and another company using that radar to make their planes undetectable).
Potential mitigations: Create and enforce rules that all users must indicate if AI was used to generate or paraphrase text. Do not allow AI tool detection outputs to be used as evidence by disputants that other parties have faked or modified their information with AI. Don't rely on these tools for organizational detection purposes unless you have proven they are highly reliable for your specific detection use cases. Never submit private information into cloud AI text detection systems. Always make sure you keep any detection tools up to date and expect them to get outdated fairly quickly. Never submit private information into cloud AI detection systems.
AI Risk 11: AI detecting AI generated or AI modified images, audio and video

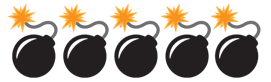
AI detecting AI generated or AI modified images, audio and video
Description: AI tools are available that claim to detect if images, videos or audio has been altered using human tools or AI tools.
AI Quality: Quite low, and maybe not even reliable enough to use as a 'second opinion' source. When it comes to making decisions or enforcing rules that evidence will not be accepted because it has been altered, you need to be certain, and these tools appear unreliable in my testing. Notice how the cloning detection on my carpet image highlighted areas that missed where the stain was reduced. My fake hole in the wall video resulted in a 89.6% confidence level it was not altered, and my altered audio with the swapped word resulted in a 99.4% confidence it was not altered.
Current threat rating: High (5 bombs). In my testing, these tools had a 100% failure rate in catching my somewhat obvious alterations (I think the video and audio should have been easily detected). They also showed outputs with statistics and visualizations that made them look credible. AI detection is based on AI outputs, which means detection tools are only available a while after new tools are released, resulting in detection tools always lagging the industry. Most of the tools I evaluated require users to submit information to the cloud which adds the additional risks of private information being submitted to the cloud servers.
Possible future risks: A goal of AI text is to be undetectable from human outputs, so smart AI developers will actually use these text detection tools as a way for training their AI to become more human like and undetectable. I see these tools as a double-edged sword where these AI detection engines are used as tools used against them to defeat their detection abilities. For example, if I was working on CGI effects in Hollywood, I would use these tools to improve the quality of my fakery.
Potential mitigations: Create and enforce rules that all users must indicate if AI was used to generate or alter images, videos or audio. Do not allow AI text detection outputs from these systems to be used as evidence that other parties have faked or modified their information with AI. Don't rely on these tools for organizational detection purposes unless you have proven they are highly reliable for your specific use cases. Never submit private information into cloud AI detection systems.
AI risks conclusion
○○ AI risk number 1 - the ability to alter, fabricate and expand information ○○
While researching AI tools, planning tests, trying technologies, analyzing results, and formulating my risk ratings, an overarching justice sector risk emerged - fair and equitable justice and dispute resolution outcomes are highly dependent on the determination of relevant facts.
AI can fabricate and alter information in ways humans, and even AI itself, can't reliably detect - effectively obfuscating the truth. AI can also quickly expand information volumes, adding further burden our overwhelmed courts, boards, panels and tribunals. For these reasons, I see AI's ability to alter, fabricate and expand information as sector risk #1.
Considering AI risks more broadly, we need to learn from our past mistakes adopting other technologies and avoid similar AI mistakes.
○○ Lessons of unintended harms from Internet and smart phone adoption ○○
We adopted the public Internet relatively quickly (lets say 12 years, 1994-2006), and the benefits have been incredible. But in adopting it, we also walked somewhat blindly into the hidden cost of 'free'. We unknowingly transitioning ourselves from the client of service providers paid to meet our needs - and into the product being sold.
The twisted incentives of the 'free' (or heavily subsidized) online services are everywhere. Companies thrive that grab our attention in negative ways (clickbait, bias reinforcement, outrage), gather and sell our information without us knowing, create confusion around inconvenient truths, and try to steer our opinions and purchases into hidden directions of those paying the bills.
We are still facing significant social costs associated to the way the Internet is structured, and AI could be used in ways that amplifies these harms... "Hey AI, make me a clickbait title and web page from a popular polarizing google search that gathers peoples personal information and maximizes them scrolling over ads".
For a second example, we rapidly adopted smart phones (lets say 8 years, 2007-2015). Of course this is also a highly beneficial technology. But here again, we walked somewhat blindly into carrying a connected device everywhere. Smart phones are proven to constantly distract us, undermine physical and mental health, amplify social isolation and loneliness, damage self esteems, and impact the cognitive development of youth - and all while gathering levels of personal information about us we wouldn't share with our closest friends. Unless we address these fundamental problems with smart phones, AI could amplify these harms too... "Hey AI, learn what this person looks at on their phone and adapt suggested content to maximize how often they check their phone and their total screen time"
The lessons of unguided technical adoption are clear. If we let powerful technologies (and companies) set their own course, we probably won't fully like where it takes us - and once we realize how they're harmful, they're too deeply established to change.
○○ AI's is incented to take your information and copy and sell what you do ○○
So, if the harms of the Internet (we're the product) and smart phones (always connected and looking at a device) grew out of misaligned human and societal incentives, does AI have any perverse incentives we should avoid?
While it doesn't apply to all AI systems, one perverse AI incentive does stand out to me. A lot of AI systems need to consume our human output to train themselves, resulting in the AI companies needing 'grey-area' thefts of information, contribution and works to achieve their goals. For example, in fields where people get their livelihoods and a sense of meaning from their creations, these AI's are incented to find ways to take their work so it can replicate and replace them (ahem, Adobe). In areas where AI is used to help corporations improve efficiency, it will be incented to absorb corporate information so that it can learn and become a similar source of corporate advantage for competitors.
To simplify this perverse incentive, I find it useful to think of these types of AI as a "supercharged information thief and copycat focused on displacing you or your services". To thrive, many AI systems have to infiltrate our work, customers, companies, and industries, and take whatever it can, from wherever it can, so that it can learn, copy and provide this service itself - and then get funded from taking the revenue from those it copied. To make this even more perverse, nobody knows how complex AI works, so it can never teach us what it does, making this theft a one way street.
You don't have to look far to see this perverse incentive in action, just google search the lawsuits by groups like record labels, newspapers, and authors guilds claiming the theft of their information by AI companies.
It is also important to read up on regional principles of privacy, copyright laws, and trade secret laws (I'm in BC, Canada) to evaluate how these AI actions align to expected human behaviors. I know these principles and laws were not written for AI, but I don't see any reason why they shouldn't be adapted to include AI. We created these principles and laws out of their importance to a high functioning social contract, and our social contracts should apply to AI too.
○○ AI's incredible power means we have to be incredibly responsible with it ○○
In closing, I see AI as one of the more powerful technologies humans have ever created - its many times more powerful and disruptive than the Internet or smart phones. Spending time focused on AI risks, I find myself agreeing with those that say its too dangerous to let it run its own course. Human history is already full of tragic technological adoption mistakes that span everything from deep water drilling to insecticides to plastics. While AI could help us find solutions to our problems, I believe it is much more likely to be aligned to maximization of our existing patterns.
We simply do not have the same margin of error we had 50 years ago. With each exponential leap in the power and reach of our technologies, the costs of our mistakes grow exponentially too. Technological change is happening faster and at larger scales, and we are already overwhelmed by many human-caused global problems that we seem unable to solve. We must find ways to do better for our own futures and the sake of future generations.
While this means we should have started defining policies and rules to mitigate AI risks years ago, the next best time to start is today. It is relatively early days for AI, so if we stay informed and attentive, openly share our concerns and ideas, and limit AI systems with the right guardrails and safeguards, I believe we can still realize many benefits from this powerful new technology - while avoiding unnecessary risks and unacceptable future costs.
I hope you enjoyed Part 1 of this series focused on AI risks, and look forward to planning, researching, and writing my next article its opportunities and benefits.
Until our next article, keep on exploring new technologies, and, where you can, take some time to share your findings and opinions so we can learn from each other and design a better future together!
Mike Harlow
Solution Architect
Hive One Justice Systems, Hive One Collaborative Systems
©2024
Where can you get more information?
If you have questions specific to this article or would like to share your thoughts or ideas with us, you can reach us through the contact form on our web site.
If you are interested in our open source feature-rich DMS system that brings advanced technology and capabilities to dispute resolution organizations, visit the DMS section of our web site.
If you want to learn more about our analysis and thoughts on Artificial Intelligence and alternative technologies, we offer live sessions and lectures that provide much greater detail. These sessions also allow breakout discussions around key areas of audience interest. To learn more about booking a discussion or lecture, please contact us through our web site.
If you are interested in other articles on advanced dispute resolution technologies, check out our designing the future blog.
If you are an organization that is seeking analysis and design services to support your own explorations into a brighter transformed future, we provide the following services:
Current state analysis: where we evaluate your existing organizational processes and systems and provide a list of priority gaps, recommendations, opportunities, and solutions for real and achievable organizational improvement.
Future state design: where we leverage our extensive technology, design, creativity, and sector experience to engage in the "what is" and "what if" discussions that will provide you with a clear, compelling and achievable future state that you can use to plan your transformation.
Comentarios